The formation of stars and planets has become one of the central areas of research in astrophysics during the past few decades, boosted to an unprecedented level by the new generation of space and ground-based observatories spanning from the X-rays to millimeter wavelengths. A number of all-sky (e.g. 2MASS, WISE, Gaia, e-ROSITA) but also numerous focused surveys and individual observations continue producing rapidly growing volumes of – increasing quality – data.
Today, we have in hand advanced computing methods and higher computing power, which allows us to apply automated and unbiased analyses to these large but often inhomogeneous datasets in order to shed light on particular aspects of the current star-formation paradigm.
Machine learning techniques find applications in a wide range of topics in astrophysics ranging from exoplanet transit detection to galaxy cluster mass estimation and the morphological classification of radio AGN. However applications of such methods in star-formation was not initiated until very recently.
In the proposed symposium we aim to focus on the applications of machine learning techniques in the field of star and planet formation. Special attention will be given to successful existing paradigms (e.g. data processing, morphological classifications and pattern detection). Since applications of machine learning in star and planet formation can still be considered an emerging field, we reserve one special block for speakers to discuss best practices and limitations from different astrophysical disciplines. We expect, thus, our symposium to not only appeal to the star and planet formation, but a much wider audience that is interested in applications of machine learning techniques. The proposed meeting is motivated from the recently launched H2020/SPACE project NEMESIS, that aims, among others, to bring Big Data techniques in the field of star and planet formation.
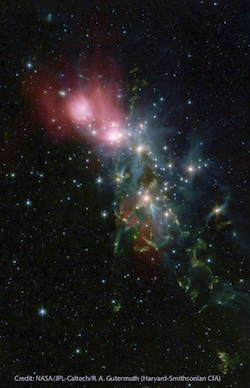
Scientific Organizers: Odysseas Dionatos (Chair) Marc Audard (co-Chair) Frederique Motte Christian Schneider
Invited speakers
- Manina Kounkel (Vanderbilt University, USA)
- Sotiria Fotopoulou (University of Bristol, UK)
- Ashish Mahabal (California Institute of Technology, USA)
- Alvaro Hacar (University of Vienna)